Diversity Is Not Enough: Why Collective Intelligence Requires Both Diversity and Disagreement
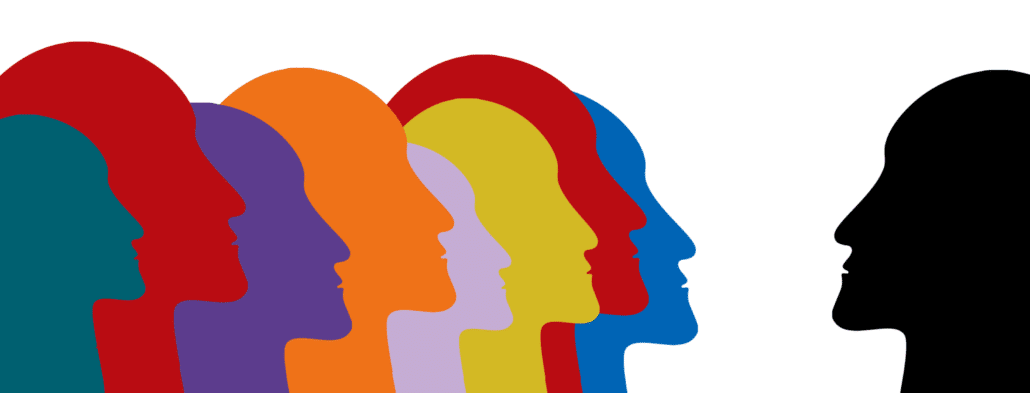
Teams are the backbone of critical institutions. Companies are run by top management teams, science is produced by research teams, and justice is rendered by teams of jurors. Our use of teams signals a commitment to the promise of diversity: More voices lead to more intelligent decisions than fewer voices do. Moreover, meta-analyses reveal the precise type of diversity that enhances collective intelligence. It’s not diversity in surface-level demographic characteristics of team members that enhances collective intelligence. It’s deep-level diversity, where team members differ in their viewpoints and information. This makes sense intuitively. Different people possess different pieces of information. Putting people together in teams gives them all the diverse pieces they need to solve complex puzzles. But teams seldom realize this promise in practice. They may have all the right pieces, but they frequently fail to connect the pieces to see the full picture.
Diversity therefore helps. But diversity alone is not enough. How can we ensure that teams use their diverse information to make more-intelligent collective decisions? This is the question my colleagues and I set out to answer in a recent study, published in IEEE Transactions on Computational Social Systems. To answer this question, we used an agent-based model: a type of computer simulation in which decision-makers known as “agents” are programmed on the basis of known psychological principles. These agents are then allowed to interact with other agents in teams across a variety of decision-making situations. Unlike laboratory studies using a limited sample of human subjects, an agent-based model lets us peer directly into the black box of team decision-making, as simulated across tens of thousands of different decision-making situations.
Leverage the wisdom of disagreement.
Our teams have five agents who must collectively decide between three alternatives, only one of which is correct. To reach a decision, teams engage in conversation, with agents taking turns sharing a piece of information with their teams and voting on a decision alternative. Because all agents possess some unique information that other members do not, information shared during conversations can change how members vote, thus bringing teams toward a consensus. The conversation continues until teams reach a consensus on the correct answer, get stuck on an incorrect answer, or reach an impasse, with agents repeatedly unable to reach a consensus.
Teams were given one of four distinct strategies for how agents should share information. Each information-sharing strategy presumes a different logic of how diversity can enhance intelligent collective decisions.
- The agreement strategy reflects a preference for affirming the opinions of others. Agents using this strategy share their piece of information that best supports the alternative that the prior agent’s information supported.
- The advocacy strategy reflects the idea that individuals enhance collective intelligence by making the strongest possible case in favor of their own interests. Agents using this strategy share their piece of information that best supports the alternative they personally prefer.
- The disagreement strategy reflects a preference to disconfirm the opinions of others. Agents using this strategy share their piece of information that best refutes the alternative that the prior agent’s information supported.
- The random strategy serves as a baseline and reflects the principle that teams benefit by generating and selectively retaining random variability. Agents using this strategy share a piece of information completely at random.
Subscribe to the Ethical Systems newsletter
We examined how these four information-sharing strategies affected two aspects of collective decision-making: accuracy (how often teams reach consensus on the correct answer) and speed (how many interactions it takes teams to reach the correct answer). Taking advantage of our ability to simulate teams across different situations, we explored how these four strategies affected decision accuracy and speed at varying levels of information load and deep-level diversity. Information load varied from 10 to 100 different pieces of information available about the situation. Deep-level diversity varied in that 25%, 50%, or 75% of the information could be unique and held by only one team member, rather than shared by all team members.
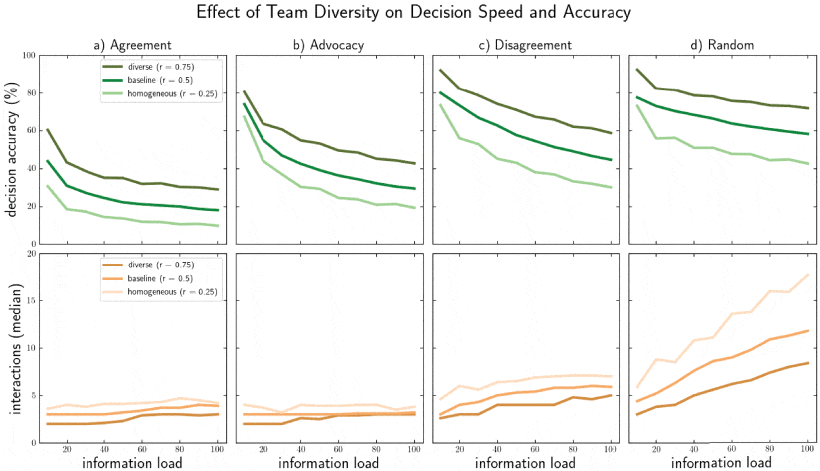
As illustrated in the figure above, our agent-based model surfaces a number of relevant insights.
- Diversity matters. Regardless of what information-sharing strategy a team used, greater deep-level diversity (where team members held more unique information and less information was held in common) was associated with more accurate decisions reached more rapidly.
- Agreement erodes intelligence. Sharing only information that agrees with other team members is a uniformly poor strategy by every metric. This finding aligns with the general lesson of groupthink: When everybody is thinking the same way, nobody is thinking much at all.
- Speed can trade off with accuracy. The random strategy leads to the most accurate decisions overall because the random sharing of information prompts teams to explore the entire space of available information. But it also has a drastic effect on speed: As the amount of information available increases, random sharing becomes prohibitively time-consuming.
- Disagreement optimally balances trade-offs. The disagreement strategy, in contrast, avoids these stark speed/accuracy trade-offs. Of all strategies, it enhances decision accuracy without becoming prohibitively time-consuming as the amount of information available increases.
Although our model identifies which information-sharing strategies teams should pursue to manage speed/accuracy trade-offs, it cannot predict what teams actually will do. Our model accounts for the fact that teams are boundedly rational, as it does not assume that team members can perfectly implement any given strategy. But our model does not account for other factors at play when actual teams use information-sharing strategies. For instance, when a team intentionally tries to implement an information-sharing strategy, “windows of opportunity” arise that allow members to engage in broader discussions about team dynamics. These broader discussions can create additional benefits that are independent of the actual strategy being implemented. Our study sets the stage for future work to explore such factors.
The bottom line of our study is that diversity matters: When team members have more unique information, they make more intelligent decisions. But diversity alone is not enough. A bad information-sharing strategy in the team can leave the important information unsaid. The most efficient and scalable approach for information-intensive decisions is to leverage the wisdom of disagreement. It is by challenging the assumptions and opinions of our team members that we produce collective intelligence, a scarce but sorely needed quality for today’s teams.
Ravi S. Kudesia is an assistant professor at the Fox School of Business, Temple University. You can follow his updates on ResearchGate.
Lead image: Gerd Altmann / Pixabay
This article was originally published on Heterodox Academy, and is reprinted with permission.